Next-Generation Video Communications
Our group works on different topics in the domain of image and video compression. In this direction, we study state-of-the-art coding standards like HEVC and we develop new methods for upcoming standards like VVC. In addition, we tackle new and unconventional approaches for coding content like medical images videos, fisheye videos, or 360°-videos.
Coding with Machine Learning
Coding with Machine Learning
Video Coding for Deep Learning-Based Machine-to-Machine Communication
Contact |
Marc Windsheimer, M.Sc. |
E-Mail: marc.windsheimer@fau.de |
Link to person |
Commonly, video codecs are designed and optimized for humans as final user. Nowadays, more and more multimedia data is transmitted for so-called machine-to-machine (M2M) applications, which means that the data is not observed by humans as the final user but successive algorithms solving several tasks. These tasks include smart industry, video surveillance, and autonomous driving scenarios. For these M2M applications, the detection rate of the successive algorithm is decisive instead of the subjective visual quality for humans. In order to evaluate the coding quality for M2M applications, we are currently focusing on neural object detection with Region-based Convolutional Neural Networks (R-CNNs).
One interesting question regarding the problem of video compression for M2M communication systems is how much the original data can be compressed until the detection rate drops. Besides, we are testing modifications on current video codecs to achieve an optimal proportion of compression and detection rate.
Deep Learning for Video Coding
Learning-based components embedded into hybrid video coding frameworks
Contact |
Simon Deniffel, M.Sc. |
E-Mail: fabian.brand@fau.de |
Link to person. |
PD Dr.-Ing. habil. Jürgen Seiler |
E-Mail: juergen.seiler@fau.de |
Link to person. |
The use of neural networks greatly increases the flexibility of video comperssion, since the underlying statistics and probability distributions can be directly learned from the data. In contrast, traditional video coders rely on hand-crafted transforms and statistics. The main topic of this research is the application of so-called conditional inter frame coders. This approach solves the problem of predicted transmission of a video frame. To exploit temporal redundancy, the coder uses a prediction signal which was generated from a previous frame. Traditionally, this prediction is subtracted from the current frame and the difference is transmitted subsequently before being added the prediction signal again on the decoder side. Using information theory, however, it can be shown that this method is not optimal. It is better to transmit the current frame under the condition of knowing the prediction. Such a conditional coder is infeasible with traditional methods. Neural networks on the other hand are able to directly learn the corresponding statistics.
We reasearch the theoretical and practical properties of a conditional inter frame coder. The focus lies on the description, modeling and alleviation of so-called information bottlenecks, which can occur in coditional coders and may reduce the achievable compression efficiency.
Residual Coder
|
Conditional Coder
|
End-to-end optimized image and video compression
Contact |
Anna Meyer, M.Sc. |
E-Mail: anna.meyer@fau.de |
Link to person |
Recently, different components of video coders have been successfully improved using neural networks. However, these approaches still rely on separately designed and optimized modules. End-to-end optimized image and video compression is an emerging field that allows optimizing the entire coding framework jointly. The most popular approaches are based on compressive autoencoders: The encoder computes a latent representation that is subsequently quantized and losslessly entropy coded. Next, the decoder computes the reconstructed image or video based on the quantized latent representation.
It has been shown that the latent space contains redundant information that can be reduced by, e.g., a spatial context model or channel conditioning. Exploiting possible remaining redundancies is challenging due to the lack of interpretability of the latent space. An interesting approach is thus designing encoder and decoder according to the lifting scheme, which results in a latent space that represents a learned wavelet decomposition. This knowledge about the structure of the latent space facilitates the development of efficient learning-based methods for image and video compression. In addition, approaches from classical wavelet compression can be adopted.
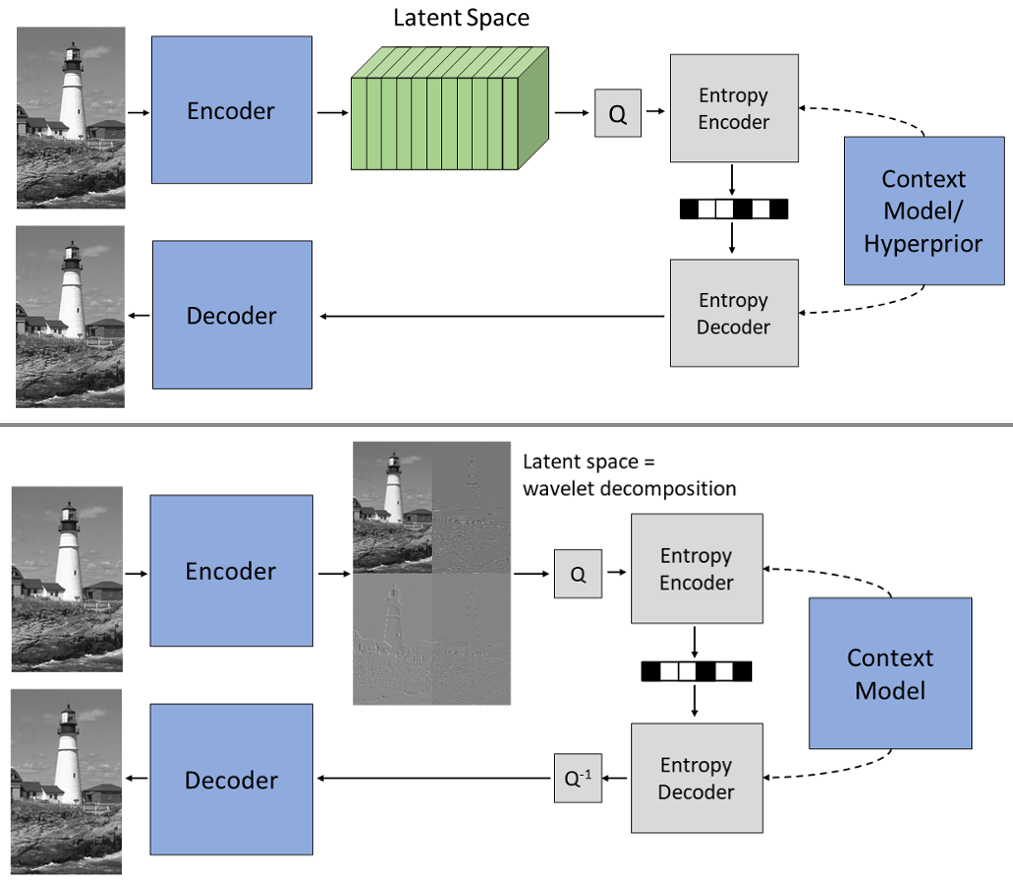
Energy and Power Efficient Video Communications
Energy and Power Efficient Video Communications
Nowadays, video communications have conquered the mass markets such that billions of end-users worldwide make use of online video applications on highly versatile devices like smartphones, TVs, or tablet PCs. Recent studies show that 1% of the greenhouse gas emissions worldwide are related to video communication services (link). This number includes all factors in the video communication toolchain such as video capture, compression, storage, transmission, decoding, and replay. Due to the large impact and the potential rise in video communication demand in the future, it is highly important to investigate the energy efficiency of practical solutions and come up with new ideas to allow a sustainable use of this technology.
To tackle this important problem, our group is committed to perform research in the field of energy efficient video communication solutions. In the past, we constructed dedicated measurement setups to be able to analyze the energy and the power consumption of various hardware and software tools being related to video communications. In terms of hardware, we tested desktop PCs, evaluation boards, smartphones, and distinct components of these devices. In terms of software, we investigated various decoders for multiple compression standards, hardware chips, and fully-functional media players. With the help of this data, we were able to develop accurate energy and power models describing the consumption in high detail. Additionally, these models allowed us to come up with new ideas to reduce and optimize the energy consumption.
We strive to dig deeper into this topic to obtain a fundamental understanding of all components contributing to the overall power consumption. Current topics include the encoding process, streaming and transmission issues, and modern video formats like 360° video coding. For future work, we are always searching for interesting ideas and collaborations to contrive new and promising solutions for energy efficient video communications. We are happy if you are interested and support our work.
Currently, we are working on the following topics:
Energy Efficient Video Coding
Contact |
Matthias Kränzler, M.Sc. |
E-Mail: matthias.kraenzler@fau.de |
Link to person |
In recent years, the amount and share of video-data in the global internet data traffic has steadily increasing. Both the encoding on the transmitter side and the decoding on the receiver side have a high energy demand. Research on energy-efficient video decoding has shown that it is possible to optimize the energy demand of the decoding process. This research area deals with the modeling of the energy required for the encoding of compressed video data. The aim of the modeling is to optimize the energy efficiency of the entire video coding.
„Big Buck Bunny“ by Big Buck Bunny is licensed under CC BY 3.0
Energy Efficient Video Decoding
Contact |
Geetha Ramasubbu, M.Sc. |
E-Mail: geetha.ramasubbu@fau.de |
Link to person |
This field of research tackles the power consumption of video decoding systems. In this respect, software as well as hardware systems are studied in high detail. An detailed analysis of the decoding energy on various platforms with various conditions can be found on the DEVISTO homepage:
Decoding Energy Visualization Tool (DEVISTO)
With the help of a high number of measurements, sophisticated energy models could be constructed which are able to accurately estimate the overall power and energy. A visualization of the modeling process for software decoding is given on the DENESTO homepage:
Decoding Energy Estimation Tool (DENESTO)
Finally, the information from the model can be exploited in rate-distortion optimization during encoding to obtain bit streams requiring less decoding energy. The source code of such an encoder can be downloaded here:
Decoding-Energy-Rate-Distortion Optimization for Video Coding (DERDO)
Energy-efficient Video Encoding
Contact |
Geetha Ramasubbu., M.Sc. |
E-Mail: geetha.ramasubbu@fau.de |
Link to person |
The research on energy consumption is useful and relevant for several reasons. Firstly, we use many portable devices, such as smartphones, or tablet PCs, to record, store, and upload videos to the Internet or streaming. A necessary step here is to compress the videos, which have a significant energy requirement and limit the battery of portable devices. Secondly, the total energy consumption of today’s coding systems is globally significant. Most video-based social networking services often use huge server farms to encode and transcode, which causes related costs due to their energy consumption. Therefore, it is beneficial for practical applications if the encoding process requires little electrical energy, as this can extend the battery life of portable devices and reduce energy consumption costs.
Furthermore, video IP traffic has increased significantly in recent years and is expected to be 82% by 2022. In addition, the compression methods used for encoding have evolved considerably in recent years. As a result, not only do the modern codecs provide a greater number of compression methods, but their processing complexity has also greatly increased, leading to a significant increase in the energy demand on the transmitter side. Therefore, research on energy-efficient video encoding is critical and globally significant. This research deals with measuring and modeling the energy consumption of various encoder systems. The encoding energy modeling aims to obtain an energy estimate of the encoding process and identify the energy-demanding encoding sub-processes.
Coding of Ultra Wide-Angle and 360° Video Data
Coding of ultra wide-angle and 360° video data
Projection-based video coding
Contact |
Andy Regensky, M.Sc. |
E-Mail: andy.regensky@fau.de |
Link to person |
Ultra-wide angle and 360° video data is subject to a variety of distortions that do not occur in conventional video data recorded with perspective lenses. These distortions occur mainly because ultra wide-angle lenses do not follow the pinhole camera model and therefore have special image characteristics. This becomes clear, for example, as straight lines are displayed in a curved form on the image sensor. This is the only way to achieve fields of view of 180° and more with only one camera. By means of so-called stitching processes, several camera views can be combined to form 360° video, which allow a complete all-round view. Often this is achieved by using two ultra wide-angle cameras, each camera capturing a hemisphere. To be able to compress the resulting spherical 360° recordings using existing video codecs, the images must be projected onto the two dimensional image surface. Various mapping functions are used for this purpose. Often, the Equirectangular format is chosen, which is comparable to the representation of the globe on a world map, and thus depicts 360° in horizontal and 180° in vertical direction.
Since conventional video codecs are not adapted to mapping functions deviating from the perspective projection, losses occur which can be reduced by taking the actual projection formats into account. Therefore, in this project different coding aspects are investigated and optimized with respect to the occurring projections of ultra wide-angle and 360° video data. A special focus lies on projection-based motion compensation and intra-prediction.
Coding of Screen Content
Coding of screen content
Screen content coding based on machine learning and statistical modelling
Contact |
Hannah Och, M.Sc. |
E-Mail: hannah.och@fau.de |
Link to person |
In recent years processing of so-called screen content has increasingly attracted attention. Screen content represents images, which can typically be seen on desktop PCs, smartphones or similar devices. Such images or sequences have very diverse statistical properties. Generally, they contain ‘synthetic’ content, namely buttons, graphics, diagrams, symbols, texts, etc. which have two significant characteristics: small varieties of colors as well as repeating patterns. Next to aforesaid structures, screen content also includes ‘natural’ content, like photographs, videos, medical images or computer generated photo-realistic animations. Unlike synthetic content natural images are characterized by irregular color gradients and a certain amount of noise. Screen content is typically a mixture of both synthetic and natural parts. The transmission of such images and image sequences is required for a multitude of applications such as screen sharing, cloud computing and gaming.
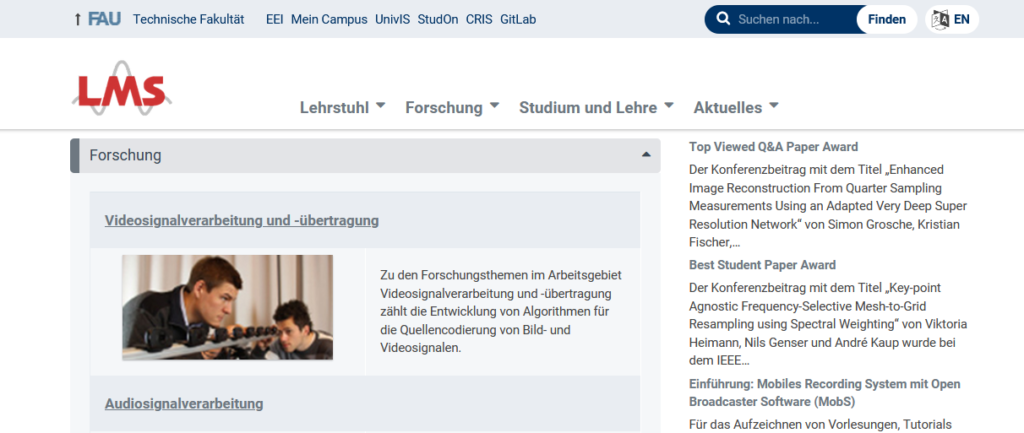
However, screen content can be a challenge for conventional coding schemes, since they are mostly optimized for camera-captured (‘natural’) scenes and cannot compress screen content efficiently. Thus, this project focuses on the further development and performance measurement based on a novel compression method for lossless and visually lossless or lossy coding of screen content images and sequences. Particular emphasis will be placed on a combination of machine learning and statistical modeling.
Coding of Point Cloud data
Coding of Point Cloud data
Coding of point cloud geometry and attributes using deep learning tools
Contact |
Dat Thanh Nguyen, M.Sc. |
E-Mail: dat.thanh.nguyen@fau.de |
Link to person |
Point Clouds are becoming one of the most common data structures to represent 3D scenes as it enables six degrees of freedom (6DoF) viewing experience. However, a typical point cloud contains millions of 3D points and requires a huge amount of storage. Hence, efficient Point Cloud Compression (PCC) methods are just inevitable in order to bring point cloud into practical applications. Unlike 2D image/video, point clouds are sparse and irregular (see the image), which make the compression task even more difficult.
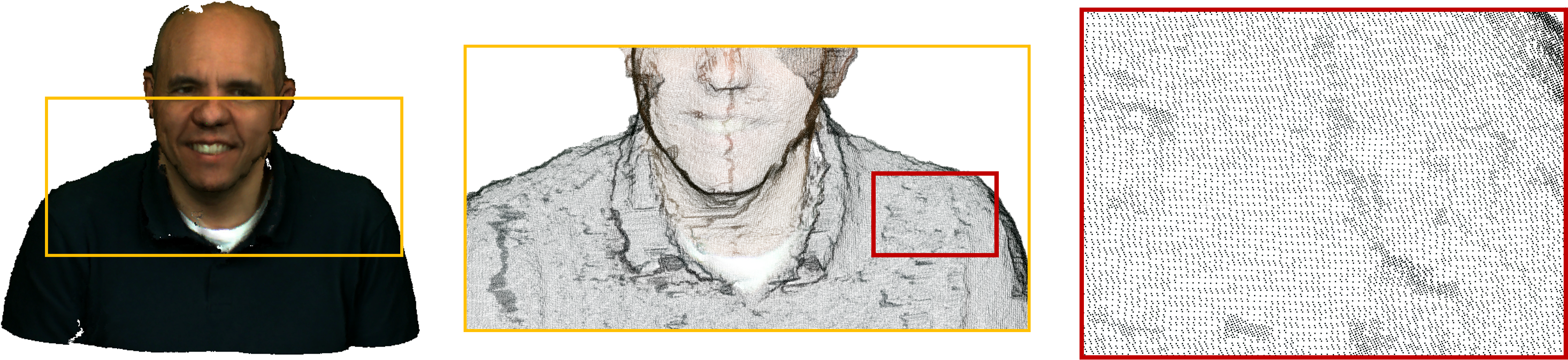
In the recent years, the research society has been paying attention on this type of data, but the compression rate is still below the compression rates of 2D-image coding algorithms (JPEG, HEVC, VVC,…). With the help of recent advances in deep learning techniques, in this project, we aim to tackle challenges in PCC including:
- Sparsity – most of the 3D space is empty, typically less than 2% of space is occupied, however, exploiting the redundancy and encoding the non-empty space are not easy tasks.
- Irregularity – unlike 2D images, where pixels are sampled uniformly over 2D planes, irregular sampling of point clouds makes it difficult to use traditional signal processing methods.
- Huge spatial volume – the information contained in a single 10 bits point cloud frame already equivalent to 1024 2D images of size 1024 × 1024. Such a point cloud would require enormous computational operations when applying any kind of signal processing technique.
Point Clouds can be encoded and then used for different purposes such as VR, world heritage, medical analysis, etc. And thus, in this project, we investigate geometry and attributes coding in both lossless and lossy scenarios to provide solutions for various applications and purposes.
Publications
2024
The Bjøntegaard Bible - Why your Way of Comparing Video Codecs May Be Wrong
In: IEEE Transactions on Image Processing 33 (2024), p. 987 - 1001
ISSN: 1057-7149
DOI: 10.1109/TIP.2023.3346695
BibTeX: Download
, , , , , , , , , , :
Analysis of Neural Video Compression Networks for 360-Degree Video Coding
Picture Coding Symposium (PCS) (Taichung, 12. June 2024 - 14. June 2024)
BibTeX: Download
, , :
Geometry-Corrected Geodesic Motion Modeling with Per-Frame Camera Motion for 360-Degree Video Compression
IEEE International Conference on Acoustics, Speech, and Signal Processing (ICASSP) (Seoul, 14. April 2024 - 19. April 2024)
DOI: 10.1109/ICASSP48485.2024.10446915
URL: https://arxiv.org/abs/2312.09266
BibTeX: Download
, :
2023
Processing Energy Modeling for Neural Network Based Image Compression
accepted for IEEE International Conference on Image Processing (ICIP) (Kuala Lumpur, 8. October 2023 - 11. October 2023)
URL: https://arxiv.org/abs/2306.16755
BibTeX: Download
, , , , :
Video Decoding Energy Reduction Using Temporal-Domain Filtering
First International Workshop on Green Multimedia Systems (GMSys '23) on the ACM Multimedia Systems (MMSys) (Vancouver, 7. June 2023 - 10. June 2023)
DOI: 10.1145/3593908.3593948
URL: https://arxiv.org/abs/2306.06917
BibTeX: Download
, , , :
Energy Efficiency in Video Compression
In: IEEE MMTC Communications - Frontiers 18 (2023), p. 8-14
Open Access: https://site.ieee.org/comsoc-mmctc/files/2023/10/MMTC-May-2023-18_3.pdf
BibTeX: Download
, , , , :
Sweet Streams are Made of This: The System Engineer’s View on Energy Efficiency in Video Communications (Preprint)
In: Ieee Circuits and Systems Magazine 23 (2023), p. 57-77
ISSN: 1531-636X
DOI: 10.1109/MCAS.2023.3234739
BibTeX: Download
, , , :
Power Reduction Opportunities on End-User Devices in Quality-Steady Video Streaming
15th International Conference on Quality of Multimedia Experience (QoMEX) (Gent, 20. June 2023 - 22. June 2023)
DOI: 10.48550/arXiv.2305.15117
URL: https://arxiv.org/abs/2305.15117
BibTeX: Download
, , , , :
Motion Plane Adaptive Motion Modeling for Spherical Video Coding in H.266/VVC
IEEE International Conference on Image Processing (ICIP) (Kuala Lumpur, 8. October 2023 - 11. October 2023)
DOI: 10.1109/ICIP49359.2023.10222661
URL: https://arxiv.org/abs/2306.13694
BibTeX: Download
, , :
2022
Evaluation of Video Coding for Machines Without Ground Truth
2022 IEEE International Conference on Acoustics, Speech and Signal Processing (ICASSP) (Singapore, 22. May 2022 - 27. May 2022)
DOI: 10.1109/ICASSP43922.2022.9747633
URL: https://arxiv.org/abs/2205.06519
BibTeX: Download
, , , , :
Rate-Distortion Optimal Transform Coefficient Selection for Unoccupied Regions in Video-Based Point Cloud Compression
In: IEEE Transactions on Circuits and Systems For Video Technology (2022), p. 1-1
ISSN: 1051-8215
DOI: 10.1109/TCSVT.2022.3185026
URL: https://arxiv.org/abs/2206.12186
BibTeX: Download
, , :
Beyond Bjontegaard: Limits of Video Compression Performance Comparisons
IEEE International Conference on Image Processing (Bordeaux, 17. October 2022 - 19. October 2022)
DOI: 10.1109/ICIP46576.2022.9897912
BibTeX: Download
, , , :
Modeling of Energy Consumption and Streaming Video QoE using a Crowdsourcing Dataset
The 14th International Conference on Quality of Multimedia Experience (QoMEX) (Lippstadt, 5. September 2022 - 7. September 2022)
DOI: 10.1109/QoMEX55416.2022.9900886
BibTeX: Download
, , , , :
Energy Efficient Video Decoding for VVC Using a Greedy Strategy Based Design Space Exploration
In: IEEE Transactions on Circuits and Systems For Video Technology 32 (2022), p. 4696-4709
ISSN: 1051-8215
DOI: 10.1109/TCSVT.2021.3130739
URL: https://arxiv.org/abs/2111.12194
BibTeX: Download
, , :
Modeling the HEVC Encoding Energy Using the Encoder Processing Time
IEEE International Conference on Image Processing (ICIP) 2022 (Bordeaux, 16. October 2022 - 19. October 2022)
DOI: 10.1109/ICIP46576.2022.9897306
URL: http://arxiv.org/abs/2207.02676
BibTeX: Download
, , :
A Bit Stream Feature-Based Energy Estimator for HEVC Software Encoding
Picture Coding Symposium (PCS 2022) (San Jose, California, 7. December 2022 - 9. December 2022)
DOI: 10.1109/PCS56426.2022.10018048
URL: https://arxiv.org/abs/2212.05609
BibTeX: Download
, , :
2021
Editorial to the Special Section on Optimized Image/Video Coding Based on Deep Learning
In: IEEE Open Journal of Circuits and Systems 2 (2021), p. 611 - 612
ISSN: 2644-1225
DOI: 10.1109/OJCAS.2021.3124408
BibTeX: Download
, , :
Robust Deep Neural Object Detection and Segmentation for Automotive Driving Scenario with Compressed Image Data
IEEE International Symposium on Circuits and Systems (ISCAS) (Daegu (Virtual Conference), 23. May 2021 - 25. May 2021)
DOI: 10.1109/ISCAS51556.2021.9401621
URL: https://arxiv.org/abs/2205.06501
BibTeX: Download
, , , :
Saliency-Driven Versatile Video Coding for Neural Object Detection
IEEE International Conference on Acoustics, Speech and Signal Processing (ICASSP) (Toronto (Virtual Conference), 6. June 2021 - 11. June 2021)
DOI: 10.1109/ICASSP39728.2021.9415048
URL: https://arxiv.org/abs/2203.05944
BibTeX: Download
, , , :
Analysis of Neural Image Compression Networks for Machine-to-Machine Communication
IEEE International Conference on Image Processing (ICIP) (Anchorage (virtual), 19. September 2021 - 22. September 2021)
DOI: 10.1109/ICIP42928.2021.9506763
URL: https://arxiv.org/abs/2205.06511
BibTeX: Download
, , , :
Optimization of Probability Distributions for Residual Coding of Screen Content
2021 IEEE International Conference on Visual Communications and Image Processing (VCIP) (München, 5. December 2021 - 8. December 2021)
DOI: 10.1109/VCIP53242.2021.9675326
URL: https://arxiv.org/abs/2212.01122
BibTeX: Download
, , :
A Novel Viewport-Adaptive Motion Compensation Technique for Fisheye Video
IEEE International Conference on Acoustics, Speech and Signal Processing (ICASSP) (Toronto, 6. June 2021 - 11. June 2021)
DOI: 10.1109/ICASSP39728.2021.9413576
URL: https://arxiv.org/abs/2202.13892
BibTeX: Download
, , :
2020
Video Coding for Machines with Feature-Based Rate-Distortion Optimization
IEEE International Workshop on Multimedia Signal Processing (MMSP) (Tampere (Virtual Conference), 21. September 2020 - 24. September 2020)
DOI: 10.1109/MMSP48831.2020.9287136
URL: https://arxiv.org/abs/2203.05890
BibTeX: Download
, , , :
On Intra Video Coding and In-loop Filtering for Neural Object Detection Networks
IEEE International Conference on Image Processing (ICIP) (Abu Dhabi (virtual Conference), 25. October 2020 - 28. October 2020)
DOI: 10.1109/ICIP40778.2020.9191023
URL: https://arxiv.org/abs/2203.05927
BibTeX: Download
, , :
Decoding Energy Optimal Video Encoding for x265
IEEE 22nd Workshop on Multimedia Signal Processing (MMSP) (Tampere, 21. September 2020 - 23. September 2020)
DOI: 10.1109/MMSP48831.2020.9287054
BibTeX: Download
, , , :
Power Modeling for Video Streaming Applications on Mobile Devices
In: IEEE Access (2020)
ISSN: 2169-3536
DOI: 10.1109/ACCESS.2020.2986580
BibTeX: Download
, , , , , :
Matched Quality Evaluation of Temporally Downsampled Videos with Non-Integer Factors
International Conference on Quality of Multimedia Experience (QoMEX) (Athlone, 26. May 2020 - 28. May 2020)
DOI: 10.1109/QoMEX48832.2020.9123084
BibTeX: Download
, , :
DENESTO: A Tool for Video Decoding Energy Estimation and Visualization
2020 IEEE International Conference on Visual Communications and Image Processing (VCIP) (Virtual Conference, 1. December 2020 - 4. December 2020)
DOI: 10.1109/VCIP49819.2020.9301877
BibTeX: Download
, , :
Decoding Energy Modeling for Versatile Video Coding
IEEE International Conference on Image Processing (ICIP) (Abu Dhabi, 25. October 2020 - 28. October 2020)
DOI: 10.1109/ICIP40778.2020.9190840
URL: https://doi.org/10.48550/arXiv.2209.10266
BibTeX: Download
, , :
A Comparative Analysis of the Time and Energy Demand of Versatile Video Coding and High Efficiency Video Coding Reference Decoders
IEEE International Workshop on Multimedia Signal Processing (MMSP) (Tampere, Finland, 21. September 2020 - 23. September 2020)
DOI: 10.1109/MMSP48831.2020.9287098
URL: https://arxiv.org/abs/2209.10283
BibTeX: Download
, , :
Graph-Based Compensated Wavelet Lifting for Scalable Lossless Coding of Dynamic Medical Data
In: IEEE Transactions on Image Processing 29 (2020), p. 2439 - 2451
ISSN: 1057-7149
DOI: 10.1109/TIP.2019.2947138
BibTeX: Download
, :
Multispectral Image Compression Based on HEVC Using Pel-Recursive Inter-Band Prediction
IEEE 22nd International Workshop on Multimedia Signal Processing (MMSP) (Tampere, 21. September 2020 - 24. September 2020)
DOI: 10.1109/MMSP48831.2020.9287132
URL: https://arxiv.org/abs/2303.05132
BibTeX: Download
, , :
FishUI: Interactive Fisheye Distortion Visualization
IEEE International Conference on Visual Communications and Image Processing (VCIP) (Macau, 1. December 2020 - 4. December 2020)
DOI: 10.1109/VCIP49819.2020.9301754
BibTeX: Download
, , :
2019
Intra Frame Prediction for Video Coding Using a Conditional Autoencoder Approach
Picture Coding Symposium (PCS) (Ningbo, 12. November 2019 - 15. November 2019)
DOI: 10.1109/PCS48520.2019.8954546
BibTeX: Download
, , :
Power Modeling for Virtual Reality Video Playback Applications
IEEE 23rd International Symposium on Consumer Technologies (ISCT) (Ancona, 19. June 2019 - 21. June 2019)
DOI: 10.1109/ISCE.2019.8901018
BibTeX: Download
, , , :
Decoding-Energy-Rate-Distortion Optimization for Video Coding
In: IEEE Transactions on Circuits and Systems For Video Technology 29 (2019), p. 171-182
ISSN: 1051-8215
DOI: 10.1109/TCSVT.2017.2771819
BibTeX: Download
, , :
Efficient Coding Of 360° Videos Exploiting Inactive Regions in Projection Formats
IEEE International Conference on Image Processing (ICIP) (Taipei, 22. September 2019 - 25. September 2019)
DOI: 10.1109/ICIP.2019.8803759
BibTeX: Download
, , , , :
Power-Efficient Video Streaming on Mobile Devices Using Optimal Spatial Scaling
IEEE International Conference on Consumer Electronics (ICCE) (Berlin, 8. September 2019 - 11. September 2019)
DOI: 10.1109/ICCE-Berlin47944.2019.8966177
BibTeX: Download
, , , :
Improving the Rate-Distortion Model of HEVC Intra by Integrating the Maximum Absolute Error
IEEE Int. Conf. on Acoustics, Speech, and Signal Processing (ICASSP) (Brighton, UK, 12. May 2019 - 17. May 2019)
DOI: 10.1109/icassp.2019.8683418
BibTeX: Download
, :
Extending Video Decoding Energy Models for 360° and HDR Video Formats in HEVC
Picture Coding Symposium (PCS) (Ningbo, 12. November 2019 - 15. November 2019)
DOI: 10.1109/PCS48520.2019.8954563
URL: https://doi.org/10.48550/arXiv.2209.10268
BibTeX: Download
, , :
Content Adaptive Wavelet Lifting for Scalable Lossless Video Coding
IEEE Int. Conf. on Acoustics, Speech, and Signal Processing (ICASSP) (Brighton, UK, 12. May 2019 - 17. May 2019)
DOI: 10.1109/icassp.2019.8682415
BibTeX: Download
, , :
Scalable Lossless Coding of Dynamic Medical CT Data Using Motion Compensated Wavelet Lifting with Denoised Prediction and Update
Picture Coding Symposium (PCS) (Ningbo, 12. November 2019 - 15. November 2019)
DOI: 10.1109/pcs48520.2019.8954530
BibTeX: Download
, , :
2018
Spectral Constrained Frequency Selective Extrapolation for Rapid Image Error Concealment
25th International Conference on Systems, Signals and Image Processing (IWSSIP) (Maribor, 20. June 2018 - 22. June 2018)
DOI: 10.1109/iwssip.2018.8439150
BibTeX: Download
, , :
Decoding Energy Estimation of an HEVC Hardware Decoder
IEEE International Symposium on Circuits and Systems (ISCAS) (Florenz, 27. May 2018 - 30. May 2018)
DOI: 10.1109/ISCAS.2018.8350964
BibTeX: Download
, :
Decoding Energy Modeling for the Next Generation Video Codec Based on JEM
Picture Coding Symposium (PCS) (San Francisco, 24. June 2018 - 27. June 2018)
DOI: 10.1109/pcs.2018.8456244
BibTeX: Download
, , :
Improving HEVC Encoding of Rendered Video Data Using True Motion Information
20th IEEE International Symposium on Multimedia (ISM) (Taichung, 10. December 2018 - 12. December 2018)
DOI: 10.1109/ism.2018.00063
URL: http://arxiv.org/abs/2309.06945
BibTeX: Download
, , , , , , :
Modeling the Energy Consumption of the HEVC Decoding Process
In: IEEE Transactions on Circuits and Systems For Video Technology 28 (2018), p. 217-229
ISSN: 1051-8215
DOI: 10.1109/TCSVT.2016.2598705
BibTeX: Download
, , , , :
Joint Optimization of Rate, Distortion, and Maximum Absolute Error for Compression of Medical Volumes Using HEVC Intra
Picture Coding Symposium (San Francisco, CA, 24. June 2018 - 27. June 2018)
DOI: 10.1109/pcs.2018.8456282
BibTeX: Download
, :
Compression of Dynamic Medical CT Data Using Motion Compensated Wavelet Lifting with Denoised Update
Picture Coding Symposium (San Francisco, CA, 24. June 2018 - 27. June 2018)
DOI: 10.1109/pcs.2018.8456262
BibTeX: Download
, , , :
A Hybrid Approach for Runtime Analysis Using a Cycle and Instruction Accurate Model
Architecture of Computing Systems (ARCS) (Braunschweig, 9. April 2018 - 12. April 2018)
In: Mladen Berekovic, Rainer Buchty, Heiko Hamann, Dirk Koch, Thilo Pionteck (ed.): 31st International Conference on Architecture of Computing Systems (ARCS) 2018
DOI: 10.1007/978-3-319-77610-1_7
URL: http://arcs2018.itec.kit.edu/
BibTeX: Download
, , , , :
2017
Scalable Near-Lossless Video Compression Based on HEVC
IEEE Visual Communications and Image Processing (VCIP) (St. Petersburg, Florida, 10. December 2017 - 13. December 2017)
DOI: 10.1109/VCIP.2017.8305068
BibTeX: Download
, :
A Low-Complexity Metric for the Estimation of Perceived Chrominance Sub-Sampling Errors in Screen Content Images
IEEE Int. Conf. on Image Processing (ICIP) (Beijing, 17. September 2017 - 20. September 2017)
DOI: 10.1109/ICIP.2017.8296878
BibTeX: Download
, , , , , :
Low-Complexity Enhancement Layer Compression for Scalable Lossless Video Coding based on HEVC
In: IEEE Transactions on Circuits and Systems For Video Technology 27 (2017), p. 1749 - 1760
ISSN: 1051-8215
DOI: 10.1109/TCSVT.2016.2556338
URL: http://ieeexplore.ieee.org/document/7457283/
BibTeX: Download
, , :
Video Decoding Energy Estimation Using Processor Events
IEEE International Conference on Image Processing (ICIP) (Beijing, 17. September 2017 - 20. September 2017)
DOI: 10.1109/ICIP.2017.8296731
BibTeX: Download
, :
Improving Mesh-Based Motion Compensation by Using Edge Adaptive Graph-Based Compensated Wavelet-Lifting for Medical Data Sets
IEEE Int. Conf. on Acoustics, Speech and Signal Processing (ICASSP) (New Orleans, LA, 5. March 2017 - 9. March 2017)
DOI: 10.1109/ICASSP.2017.7952408
BibTeX: Download
, :
2016
Fast CU Split Decisions for HEVC Inter Coding Using Support Vector Machines
Picture Coding Symposium (PCS) (Nuremberg, 4. December 2016 - 7. December 2016)
DOI: 10.1109/PCS.2016.7906358
BibTeX: Download
, , :
Fast Exclusion of Angular Intra Prediction Modes in HEVC Using Reference Sample Variance
IEEE International Symposium on Circuits and Systems (ISCAS) (Montréal, 22. May 2016 - 25. May 2016)
DOI: 10.1109/ISCAS.2016.7539144
BibTeX: Download
, :
Two-Stage Exclusion of Angular Intra Prediction Modes for Fast Mode Decision in HEVC
IEEE International Conference on Image Processing (ICIP) (Phoenix, AZ, 25. September 2016 - 28. September 2016)
DOI: 10.1109/ICIP.2016.7532413
BibTeX: Download
, , :
Joint Optimization of Rate, Distortion, and Decoding Energy for HEVC Intraframe Coding
IEEE International Conference on Image Processing (ICIP) (Phoenix, Arizona, 25. September 2016 - 28. September 2016)
DOI: 10.1109/ICIP.2016.7532416
URL: http://arxiv.org/abs/2203.01765
BibTeX: Download
, :
Multi-Objective Design Space Exploration for the Optimization of the HEVC Mode Decision Process
Picture Coding Symposium(PCS) (Nürnberg, 4. December 2016 - 7. December 2016)
In: Picture Coding Symposium (PCS) 2016
DOI: 10.1109/PCS.2016.7906327
URL: http://arxiv.org/abs/2203.01782
BibTeX: Download
, , , , :
A Bitstream Feature Based Model for Video Decoding Energy Estimation
Picture Coding Symposium (PCS) (Nürnberg, 4. December 2016 - 7. December 2016)
DOI: 10.1109/PCS.2016.7906400
URL: https://doi.org/10.48550/arXiv.2204.10151
BibTeX: Download
, , , , :
Graph-Based Compensated Wavelet Lifting for 3-D+t Medical CT Data
Picture Coding Symposium (Nuremberg, 4. December 2016 - 7. December 2016)
DOI: 10.1109/PCS.2016.7906385
BibTeX: Download
, :
Analysis and Exploitation of CTU-Level Parallelism in the HEVC Mode Decision Process Using Actor-based Modeling
Architecture of Computing Systems (ARCS) (Nürnberg, 4. April 2016 - 7. April 2016)
In: Springer (ed.): In Proceedings of the International Conference on Architecture of Computing Systems (ARCS), Berlin; Heidelberg: 2016
DOI: 10.1007/978-3-319-30695-7_20
BibTeX: Download
, , , , :
Probability Distribution Estimation for Autoregressive Pixel-Predictive Image Coding
In: IEEE Transactions on Image Processing 25 (2016), p. 1382-1395
ISSN: 1057-7149
DOI: 10.1109/TIP.2016.2522339
BibTeX: Download
, , , :
2015
Fast intra mode decision in HEVC using early distortion estimation
IEEE China Summit and International Conference on Signal and Information Processing, ChinaSIP 2015 (Chengdu, 12. July 2015 - 15. July 2015)
DOI: 10.1109/ChinaSIP.2015.7230465
BibTeX: Download
, :
Estimating The HEVC Decoding Energy Using High-Level Video Features
European Signal Processing Conference (EUSIPCO) (Nice, 31. August 2015 - 4. September 2015)
In: Proc. of European Signal Processing Conference (EUSIPCO) 2015
DOI: 10.1109/EUSIPCO.2015.7362652
BibTeX: Download
, :
Estimation of Non-functional Properties for Embedded Hardware with Application to Image Processing
22nd Reconfigurable Architectures Workshop (RAW) on the 29th Annual International Parallel & Distributed Processing Symposium (IPDPS) (Hyderabad, 25. May 2015 - 29. May 2015)
DOI: 10.1109/IPDPSW.2015.58
URL: http://arxiv.org/abs/2203.01771
BibTeX: Download
, , , , , :
Estimating the HEVC Decoding Energy Using the Decoder Processing Time
IEEE Int. Symp. on Circuits and Systems (ISCAS) (Lisbon, 24. May 2015 - 27. May 2015)
In: Proc. of IEEE Int. Symp. on Circuits and Systems (ISCAS) 2015
DOI: 10.1109/ISCAS.2015.7168683
URL: http://arxiv.org/abs/2203.01767
BibTeX: Download
, , :
2014
Estimating Video Decoding Energies And Processing Times Utilizing Virtual Hardware
3PMCES Workshop. Design, Automation & Test in Europe (DATE) (Dresden, 24. March 2014 - 28. March 2014)
BibTeX: Download
, , , , :
Coding of Distortion-Corrected Fisheye Video Sequences Using H.265/HEVC
IEEE International Conference on Image Processing (ICIP) (Paris, 27. October 2014 - 30. October 2014)
In: Proc. of IEEE International Conference on Image Processing (ICIP) 2014
DOI: 10.1109/ICIP.2014.7025839
BibTeX: Download
, :
Sample-based Weighted Prediction for Lossless Enhancement Layer Coding in SHVC
IEEE International Conference on Image Processing (ICIP) (Paris, 27. October 2014 - 30. October 2014)
DOI: 10.1109/ICIP.2014.7025742
BibTeX: Download
, , :
Analysis of prediction algorithms for residual compression in a lossy to lossless scalable video coding system based on HEVC
Applications of Digital Image Processing XXXVII (San Diego, CA, 17. August 2014 - 21. August 2014)
DOI: 10.1117/12.2061597
BibTeX: Download
, , :
Modeling the Energy Consumption of HEVC P- and B-Frame Decoding
Intl. Conference on Image Processing (ICIP) (Paris, 27. October 2014 - 30. October 2014)
In: Proc. of Intl. Conf. on Image Processing (ICIP) 2014
DOI: 10.1109/ICIP.2014.7025743
BibTeX: Download
, , :
Multiple description coding with randomly and uniformly offset quantizers
In: IEEE Transactions on Image Processing 23 (2014), p. 582-595
ISSN: 1057-7149
DOI: 10.1109/TIP.2013.2288928
BibTeX: Download
, , , , , :
3-D mesh compensated wavelet lifting for 3-D+t medical CT data
IEEE International Conference on Image Processing (Paris, 27. October 2014 - 30. October 2014)
DOI: 10.1109/ICIP.2014.7025737
BibTeX: Download
, , , , :
Efficient lossless coding of highpass bands from block-based motion compensated wavelet lifting using JPEG 2000
IEEE Int. Conf. on Visual Communications and Image Processing (VCIP) (Valletta, 7. December 2014 - 10. December 2014)
DOI: 10.1109/VCIP.2014.7051590
BibTeX: Download
, , , , :
Open source HEVC analyzer for rapid prototyping (HARP)
IEEE International Conference on Image Processing (Paris, 27. October 2014 - 30. October 2014)
DOI: 10.1109/ICIP.2014.7025443
BibTeX: Download
, , , , , :
In-loop noise-filtered prediction for high efficiency video coding
In: IEEE Transactions on Circuits and Systems For Video Technology 24 (2014), p. 1142-1155
ISSN: 1051-8215
DOI: 10.1109/TCSVT.2014.2302377
BibTeX: Download
, , , , :
2013
Sample-based Weighted Prediction for Lossless Enhancement Layer Coding in HEVC
Grand Challenge at Picture Coding Symposium (PCS) (San José, CA, 8. December 2013)
BibTeX: Download
, , :
Modeling the energy consumption of HEVC intra decoding
2013 20th International Conference on Systems, Signals and Image Processing, IWSSIP 2013 (Bucharest, 7. July 2013 - 9. July 2013)
DOI: 10.1109/IWSSIP.2013.6623457
URL: http://arxiv.org/abs/2203.01755
BibTeX: Download
, , , :
Multiple description coding with randomly offset quantizers
2013 IEEE International Symposium on Circuits and Systems, ISCAS 2013 (Beijing, 19. May 2013 - 23. May 2013)
DOI: 10.1109/ISCAS.2013.6571832
BibTeX: Download
, , , , , :
M-channel multiple description coding based on uniformly offset quantizers with optimal deadzone
2013 38th IEEE International Conference on Acoustics, Speech, and Signal Processing, ICASSP 2013 (Vancouver, BC, 26. May 2013 - 31. May 2013)
DOI: 10.1109/ICASSP.2013.6638009
BibTeX: Download
, , , , , :
Spiral search based fast rotation estimation for efficient HEVC compression of navigation video sequences
2013 Picture Coding Symposium, PCS 2013 (San Jose, CA, 8. December 2013 - 11. December 2013)
DOI: 10.1109/PCS.2013.6737718
BibTeX: Download
, , , , :
Motion vector analysis based homography estimation for efficient HEVC compression of 2D and 3D navigation video sequences
2013 20th IEEE International Conference on Image Processing, ICIP 2013 (Melbourne, VIC, 15. September 2013 - 18. September 2013)
DOI: 10.1109/ICIP.2013.6738359
BibTeX: Download
, , , :
Robust Rotational Motion Estimation for efficient HEVC compression of 2D and 3D navigation video sequences
2013 38th IEEE International Conference on Acoustics, Speech, and Signal Processing, ICASSP 2013 (Vancouver, BC, 26. May 2013 - 31. May 2013)
DOI: 10.1109/ICASSP.2013.6637877
BibTeX: Download
, , , :
Near-lossless compression of computed tomography images using predictive coding with distortion optimization
SPIE Medical Imaging 2013: Image Processing (Lake Buena Vista, Florida, 9. February 2013 - 14. February 2013)
DOI: 10.1117/12.2006931
BibTeX: Download
, , , :
Volumetric deformation compensation in CUDA for coding of dynamic cardiac images
2013 Picture Coding Symposium, PCS 2013 (San Jose, CA, 8. December 2013 - 11. December 2013)
DOI: 10.1109/PCS.2013.6737715
BibTeX: Download
, , , , :
Massively parallel lossless compression of medical images using least-squares prediction and arithmetic coding
2013 20th IEEE International Conference on Image Processing, ICIP 2013 (Melbourne, VIC, 15. September 2013 - 18. September 2013)
DOI: 10.1109/ICIP.2013.6738346
BibTeX: Download
, , , , :
Formangepasste diskrete Cosinus Transformation für die Prädiktionsverbesserung im HEVC
15. ITG-Fachtagung für Elektronische Medien (Dortmund, 26. February 2013 - 27. February 2013)
In: 15. ITG-Fachtagung für Elektronische Medien, Dortmund, Deutschland: 2013
DOI: 10.17877/DE290R-14782
BibTeX: Download
, , :
Pixel-based averaging predictor for HEVC lossless coding
2013 20th IEEE International Conference on Image Processing, ICIP 2013 (Melbourne, VIC, 15. September 2013 - 18. September 2013)
DOI: 10.1109/ICIP.2013.6738372
BibTeX: Download
, , , , :
Sample-based weighted prediction with directional template matching for HEVC lossless coding
2013 Picture Coding Symposium, PCS 2013 (San Jose, CA, 8. December 2013 - 11. December 2013)
DOI: 10.1109/PCS.2013.6737744
BibTeX: Download
, , , , :
2012
Optimizing frame structure with real-time computation for interactive multiview video streaming
2012 3DTV-Conference: The True Vision - Capture, Transmission and Display of 3D Video, 3DTV-CON 2012 (Zürich, 15. October 2012 - 17. October 2012)
DOI: 10.1109/3DTV.2012.6365460
BibTeX: Download
, , , :
Analysis of Mesh-Based Motion Compensation in Wavelet Lifting of Dynamical 3-D+t CT Data
IEEE International Workshop on Multimedia Signal Processing (Banff, 17. September 2012 - 19. September 2012)
DOI: 10.1109/MMSP.2012.6343432
BibTeX: Download
, , , :
On the influence of clipping in lossless predictive and wavelet coding of noisy images
29th Picture Coding Symposium, PCS 2012 (Krakow, 7. May 2012 - 9. May 2012)
DOI: 10.1109/PCS.2012.6213323
BibTeX: Download
, , , :
Analysis of displacement compensation methods for wavelet lifting of medical 3-D thorax CT volume data
2012 IEEE Visual Communications and Image Processing, VCIP 2012 (San Diego, CA, 27. November 2012 - 30. November 2012)
DOI: 10.1109/VCIP.2012.6410751
BibTeX: Download
, , , :
Compression of 2D Navigation Views with Rotational and Translational Motion
Visual Information Processing and Communication III (San Francisco, CA, 22. January 2012)
DOI: 10.1117/12.911990
BibTeX: Download
, , , :
Compression of 2D and 3D navigation video sequences using skip mode masking of static areas
29th Picture Coding Symposium, PCS 2012 (Krakow, 7. May 2012 - 9. May 2012)
DOI: 10.1109/PCS.2012.6213352
BibTeX: Download
, , , :
Representation of deformable motion for compression of dynamic cardiac image data
Medical Imaging 2012: Image Processing (San Diego, CA, 4. February 2012 - 9. February 2012)
In: Proceedings of SPIE - The International Society for Optical Engineering 8314 2012
DOI: 10.1117/12.911276
BibTeX: Download
, , , :
Mode adaptive reference frame denoising for high fidelity compression in HEVC
2012 IEEE Visual Communications and Image Processing, VCIP 2012 (San Diego, CA, 27. November 2012 - 30. November 2012)
DOI: 10.1109/VCIP.2012.6410777
BibTeX: Download
, , , :
2011
Methods and tools for wavelet-based scalable multiview video coding
In: IEEE Transactions on Circuits and Systems For Video Technology 21 (2011), p. 113-126
ISSN: 1051-8215
DOI: 10.1109/TCSVT.2011.2105552
BibTeX: Download
, , :
Analysis of Inter-Layer Prediction and Hierarchical Prediction Structures in Scalable Video Coding
In: IEEE Transactions on Broadcasting 57 (2011), p. 66-74
ISSN: 0018-9316
DOI: 10.1109/TBC.2010.2082370
BibTeX: Download
, , , :
Reusing the H.264/AVC deblocking filter for efficient spatio-temporal prediction in video coding
36th IEEE International Conference on Acoustics, Speech, and Signal Processing, ICASSP 2011 (Prague, 22. May 2011 - 27. May 2011)
DOI: 10.1109/ICASSP.2011.5946587
URL: https://arxiv.org/pdf/2207.01210.pdf
BibTeX: Download
, :
Sparse representation of dense motion vector fields for lossless compression of 4-D medical CT data
19th European Signal Processing Conference, EUSIPCO 2011 (Barcelona, 30. August 2011 - 2. September 2011)
DOI: 10.5281/zenodo.42439
BibTeX: Download
, , , :
Adaptive in-loop noise-filtered prediction for high efficiency video coding
3rd IEEE International Workshop on Multimedia Signal Processing, MMSP 2011 (Hangzhou, 17. October 2011 - 19. October 2011)
DOI: 10.1109/MMSP.2011.6093773
BibTeX: Download
, , , , :
Efficient coding of video sequences by non-local in-loop denoising of reference frames
2011 18th IEEE International Conference on Image Processing, ICIP 2011 (Brussels, 11. September 2011 - 14. September 2011)
DOI: 10.1109/ICIP.2011.6115648
BibTeX: Download
, , , , :
2010
Adaptive quantization parameter cascading for hierarchical video coding
2010 IEEE International Symposium on Circuits and Systems: Nano-Bio Circuit Fabrics and Systems, ISCAS 2010 (Paris, 30. May 2010 - 2. June 2010)
DOI: 10.1109/ISCAS.2010.5537584
BibTeX: Download
, , , :
Multiple selection approximation for improved spatio-temporal prediction in video coding
2010 IEEE International Conference on Acoustics, Speech, and Signal Processing, ICASSP 2010 (Dallas, TX, 14. March 2010 - 19. March 2010)
DOI: 10.1109/ICASSP.2010.5495253
URL: https://arxiv.org/abs/2207.01207
BibTeX: Download
, :
Spatio-temporal prediction in video coding by non-local means refined motion compensation
28th Picture Coding Symposium, PCS 2010 (Nagoya, 8. December 2010 - 10. December 2010)
DOI: 10.1109/PCS.2010.5702497
URL: https://arxiv.org/abs/2207.09729
BibTeX: Download
, , :
In-loop denoising of reference frames for lossless coding of noisy image sequences
2010 17th IEEE International Conference on Image Processing, ICIP 2010 (Hong Kong, 26. September 2010 - 29. September 2010)
DOI: 10.1109/ICIP.2010.5654136
BibTeX: Download
, , , :
Analysis of in-loop denoising in lossy transform coding
28th Picture Coding Symposium, PCS 2010 (Nagoya, 8. December 2010 - 10. December 2010)
DOI: 10.1109/PCS.2010.5702584
BibTeX: Download
, , , , :
2009
Analysis on Spatial Scalable Multiview Video Coding with Wavelets
2009 IEEE International Workshop on Multimedia Signal Processing, MMSP '09 (Rio De Janeiro, 5. October 2009 - 7. October 2009)
DOI: 10.1109/MMSP.2009.5293340
BibTeX: Download
, :
Optimized Anisotropic Spatial Transforms for Wavelet-Based Scalable Multi-View Video Coding
Visual Communications and Image Processing 2009 (San Jose, CA, 18. January 2009 - 22. January 2009)
DOI: 10.1117/12.807250
BibTeX: Download
, , :
Lagrange multiplier selection for rate-distortion optimization in SVC
2009 Picture Coding Symposium, PCS 2009 (Chicago, IL, 6. May 2009 - 8. May 2009)
DOI: 10.1109/PCS.2009.5167399
BibTeX: Download
, , , :
Model based analysis for quantization parameter cascading in hierarchical video coding
2009 IEEE International Conference on Image Processing, ICIP 2009 (Cairo, 7. November 2009 - 10. November 2009)
DOI: 10.1109/ICIP.2009.5414354
BibTeX: Download
, , , :
One-Pass Multi-Layer Rate-Distortion Optimization for H.264/SVC Quality Scalable Video Coding
2009 IEEE International Conference on Acoustics, Speech, and Signal Processing, ICASSP 2009 (Taipei, 19. April 2009 - 24. April 2009)
URL: https://www.scopus.com/inward/record.uri?partnerID=HzOxMe3b&scp=70349459711∨igin=inward
BibTeX: Download
, , , :
One-Pass Frame Level Budget Allocation Based on inter-frame Dependency
2009 IEEE International Workshop on Multimedia Signal Processing, MMSP '09 (Rio De Janeiro, 5. October 2009 - 7. October 2009)
DOI: 10.1109/MMSP.2009.5293245
BibTeX: Download
, , :
Efficient one-pass frame level rate control for H.264/AVC
In: Journal of Visual Communication and Image Representation 20 (2009), p. 585-594
ISSN: 1047-3203
DOI: 10.1016/j.jvcir.2009.09.001
BibTeX: Download
, , :
Laplace distribution based Lagrangian rate distortion optimization for hybrid video coding
In: IEEE Transactions on Circuits and Systems For Video Technology 19 (2009), p. 193-205
ISSN: 1051-8215
DOI: 10.1109/TCSVT.2008.2009255
BibTeX: Download
, , , :
Spatio-temporal prediction in video coding by best approximation
2009 Picture Coding Symposium, PCS 2009 (Chicago, IL, 6. May 2009 - 8. May 2009)
DOI: 10.1109/PCS.2009.5167407
URL: https://arxiv.org/abs/2207.09727
BibTeX: Download
, , :
Lossy compression of floating point High Dynamic Range images using JPEG2000
Visual Communications and Image Processing 2009 (San Jose, CA, 18. January 2009 - 22. January 2009)
DOI: 10.1117/12.805315
BibTeX: Download
, :
2008
Wavelet-based multi-view video coding with joint best basis wavelet packets
2008 IEEE International Conference on Image Processing, ICIP 2008 (San Diego, CA, 12. October 2008 - 15. October 2008)
DOI: 10.1109/ICIP.2008.4711984
BibTeX: Download
, , , :
On the Efficiency of Inter-Layer Prediction in H.264/AVC Quality Scalable Video Coding
IEEE International Symposium on Recent Advances in Communication Engineering (, 20. December 2008 - 23. December 2008)
BibTeX: Download
, , , :
Rate-distortion optimized frame level rate control for H.264/AVC
16th European Signal Processing Conference, EUSIPCO 2008 (Lausanne, 25. August 2008 - 29. August 2008)
URL: https://www.scopus.com/inward/record.uri?partnerID=HzOxMe3b&scp=84863745721∨igin=inward
BibTeX: Download
, , , :
Analysis of Compression of 4D Volumetric Medical Image Datasets Using Multi-View Video Coding Methods
Mathematics of Data/Image Pattern Recognition, Compression, and Encryption with Applications XI (San Diego, CA, 10. August 2008 - 14. August 2008)
DOI: 10.1117/12.794483
BibTeX: Download
, :
Analysis of spatio-temporal prediction methods in 4D volumetric medical image datasets
2008 IEEE International Conference on Multimedia and Expo, ICME 2008 (Hannover, 23. June 2008 - 26. June 2008)
DOI: 10.1109/ICME.2008.4607487
BibTeX: Download
, :
Spatial scalable JPEG2000 transcoding and tracking of regions of interest for video surveillance
13th International Fall Workshop Vision, Modeling, and Visualization 2008, VMV 2008 (Konstanz, 8. October 2008 - 10. October 2009)
URL: https://www.scopus.com/inward/record.uri?partnerID=HzOxMe3b&scp=84881575723∨igin=inward
BibTeX: Download
, :
Spatio-temporal prediction in video coding by spatially refined motion compensation
2008 IEEE International Conference on Image Processing, ICIP 2008 (San Diego, CA, 12. October 2008 - 15. October 2008)
DOI: 10.1109/ICIP.2008.4712373
URL: https://arxiv.org/abs/2207.03766
BibTeX: Download
, :
2007
Fast Video Transcoding from H.263 To H. 264/AVC
In: Multimedia Tools and Applications 35 (2007), p. 127-146
ISSN: 1380-7501
DOI: 10.1007/s11042-007-0126-7
BibTeX: Download
, , :
Complexity evaluation of random access to coded multi-view video data
15th European Signal Processing Conference, EUSIPCO 2007 (Poznan, 3. September 2007 - 7. September 2007)
URL: https://www.scopus.com/inward/record.uri?partnerID=HzOxMe3b&scp=80051659180∨igin=inward
BibTeX: Download
, :
H.263 to H.264 transconding using data mining
14th IEEE International Conference on Image Processing, ICIP 2007 (San Antonio, Texas, 16. September 2007 - 19. September 2007)
DOI: 10.1109/ICIP.2007.4379959
BibTeX: Download
, , , , , :
Wavelet-based multi-view video coding with full scalability and illumination compensation
15th ACM International Conference on Multimedia, MM'07 (Augsburg, Bavaria, 24. September 2007 - 29. September 2007)
DOI: 10.1145/1291233.1291402
BibTeX: Download
, , :
Inter-scale prediction of motion information for a wavelet-based scalable video coder
26th Picture Coding Symposium, PCS 2007 (Lisbon, 7. November 2007 - 9. November 2007)
URL: https://www.scopus.com/inward/record.uri?partnerID=HzOxMe3b&scp=84898062563∨igin=inward
BibTeX: Download
, :
Wavelet-based multi-view video coding with spatial scalability
2007 IEEE 9Th International Workshop on Multimedia Signal Processing, MMSP 2007 (Chania, Crete, 1. October 2007 - 3. October 2007)
DOI: 10.1109/MMSP.2007.4412906
BibTeX: Download
, :
Advanced Lagrange multiplier selection for hybrid video coding
IEEE International Conference onMultimedia and Expo, ICME 2007 (Beijing, 2. July 2007 - 5. July 2007)
URL: https://www.scopus.com/inward/record.uri?partnerID=HzOxMe3b&scp=46449128611∨igin=inward
BibTeX: Download
, , , :
Adaptive lagrange multiplier selection for intra-frame video coding
2007 IEEE International Symposium on Circuits and Systems, ISCAS 2007 (New Orleans, LA, 27. May 2007 - 30. May 2007)
DOI: 10.1109/ISCAS.2007.378542
BibTeX: Download
, , :
Extended lagrange multiplier selection for hybrid video coding using interframe correlation
26th Picture Coding Symposium, PCS 2007 (Lisbon, 7. November 2007 - 9. November 2007)
URL: https://www.scopus.com/inward/record.uri?partnerID=HzOxMe3b&scp=84898070973∨igin=inward
BibTeX: Download
, , , :
2006
Overview of low-complexity video transcoding from H.263 to H.264
2006 IEEE International Conference on Multimedia and Expo, ICME 2006 (Toronto, ON, 9. October 2006 - 12. October 2006)
DOI: 10.1109/ICME.2006.262547
BibTeX: Download
, , :
A new algorithm for reducing the requantization loss in video transcoding
14th European Signal Processing Conference, EUSIPCO 2006 (Florence, 4. September 2006 - 8. September 2006)
URL: https://www.scopus.com/inward/record.uri?partnerID=HzOxMe3b&scp=84862633686∨igin=inward
BibTeX: Download
, , :
Low-complexity transcoding of inter coded video frames from H.264 to H.263
2006 IEEE International Conference on Image Processing, ICIP 2006 (Atlanta, GA, 8. October 2006 - 11. October 2006)
DOI: 10.1109/ICIP.2006.312532
BibTeX: Download
, , , :
Improving the prediction efficiency for multi-view video coding using histogram matching
25th PCS: Picture Coding Symposium 2006, PCS2006 (Beijing, 24. April 2006 - 26. April 2006)
URL: https://www.scopus.com/inward/record.uri?partnerID=HzOxMe3b&scp=34047168689∨igin=inward
BibTeX: Download
, , :
Depth map compression for unstructured lumigraph rendering
Visual Communications and Image Processing (VCIP 2006) (San Jose, CA, 15. January 2006 - 19. January 2006)
DOI: 10.1117/12.642803
BibTeX: Download
, , , :
4D scalable multi-view video coding using disparity compensated view filtering and motion compensated temporal filtering
2006 IEEE 8th Workshop on Multimedia Signal Processing, MMSP 2006 (Victoria, BC, 3. October 2006 - 6. October 2006)
DOI: 10.1109/MMSP.2006.285268
BibTeX: Download
, , , :
Analysis of Multi-Reference Block Matching for Multi-View Video Coding
7th Workshop Digital Broadcasting (Erlangen, 14. September 2006 - 15. September 2006)
BibTeX: Download
, :
BeTrIS - An Index System for MPEG-7 Streams
In: EURASIP J APPL SIG P 2006 (2006), p. 1-11
ISSN: 1110-8657
DOI: 10.1155/ASP/2006/15482
BibTeX: Download
, , , :
Gradient intra prediction for coding of computer animated video
2006 IEEE 8th Workshop on Multimedia Signal Processing, MMSP 2006 (Victoria, BC, 3. October 2006 - 6. October 2006)
DOI: 10.1109/MMSP.2006.285267
BibTeX: Download
, , :
2005
On requantization in intra-frame video transcoding with different transform block sizes
2005 IEEE 7th Workshop on Multimedia Signal Processing, MMSP 2005 (Shanghai, 30. October 2005 - 2. November 2005)
DOI: 10.1109/MMSP.2005.248669
BibTeX: Download
, , :
H.264/AVC-compatible coding of dynamic light fields using transposed picture ordering
13th European Signal Processing Conference, EUSIPCO 2005 (Antalya, 4. September 2005 - 8. September 2005)
URL: https://www.scopus.com/inward/record.uri?partnerID=HzOxMe3b&scp=34247167330∨igin=inward
BibTeX: Download
, :
Statistical Analysis of Multi-Reference Block Matching for Dynamic Light Field Coding
10th International Fall Workshop - Vision, Modeling, and Visualization (VMV) (Erlangen, 16. November 2005 - 18. November 2005)
BibTeX: Download
, :
2004
Fast transcoding of intra frames between H.263 and H.264
2004 International Conference on Image Processing, ICIP 2004 (Singapore, 24. October 2004 - 27. October 2004)
DOI: 10.1109/ICIP.2004.1421682
BibTeX: Download
, , :
A fast H.263 to H.264 inter-frame transcoder with motion vector refinement
Picture Coding Symposium 2004 (San Francisco, CA, 15. December 2004 - 17. December 2004)
URL: https://www.scopus.com/inward/record.uri?partnerID=HzOxMe3b&scp=18144406177&origin=inward
BibTeX: Download
, , , :
Error control and concealment of JPEG2000 coded image data in error prone environments
Picture Coding Symposium 2004 (San Francisco, CA, 15. December 2004 - 17. December 2004)
URL: https://www.scopus.com/inward/record.uri?partnerID=HzOxMe3b&scp=18144396226&origin=inward
BibTeX: Download
, , :
Serially Connected Channels: Capacity and Video Streaming Application Scenario for Separate and Joint Channel Coding
5th International ITG Conference on Source and Channel Coding (SCC) (Erlangen, 14. January 2004 - 16. January 2004)
URL: https://www.scopus.com/inward/record.uri?partnerID=HzOxMe3b&scp=1642574168&origin=inward
BibTeX: Download
, , :
2002
Multimedia Messaging mit MPEG-7
In: Fachzeitschrift für Fernsehen, Film und elektronische Medien 56 (2002), p. 27-30
ISSN: 1430-9947
BibTeX: Download
, , :
An MPEG-7 tool for compression and streaming of XML data
2002 IEEE International Conference on Multimedia and Expo, ICME 2002 (, 25. August 2002 - 29. August 2002)
DOI: 10.1109/ICME.2002.1035833
BibTeX: Download
, , , , :
2001
Multimedia Messaging Technologien auf der Basis von MPEG-7
In: ITG-Fachbericht (2001), p. 157-162
ISSN: 0932-6022
BibTeX: Download
, , :
Adaptive Multimedia Messaging: Application Scenario and Technical Challenges
Wireless World Research Forum (Munich, 6. March 2001 - 7. March 2001)
BibTeX: Download
, , :
MPEG-Standards: Techniken und Entwicklungstrends
In: Fachzeitschrift für Fernsehen, Film und elektronische Medien 55 (2001), p. 352-362
ISSN: 1430-9947
BibTeX: Download
:
2000
A Simple Multiple Video Objects Rate Control Algorithm for MPEG-4 Real-Time Applications
3. ITG Conference on Source and Channel Coding, ITG-Fachbericht 159 (Munich, 17. January 2000 - 19. January 2000)
BibTeX: Download
, :
1999
Object-Based Texture Coding of Moving Video in MPEG-4
In: IEEE Transactions on Circuits and Systems For Video Technology 9 (1999), p. 5-15
ISSN: 1051-8215
BibTeX: Download
:
Performance and Complexity Analysis of Rate Constrained Motion Estimation in MPEG-4
In: Proceedings Multimedia Systems and Applications II, SPIE 1999
BibTeX: Download
, :
Leistungsfähigkeit und Komplexität von ratengesteuerter Bewegungsschätzung in MPEG-4
Multimedia: Anwendungen, Technologie, Systeme, ITG-Fachbericht 156 (, 27. September 1999 - 29. September 1999)
BibTeX: Download
, :
1998
Der MPEG-4 Multimedia-Standard und seine Anwendungen im MINT-Projekt
In: Der Fernmelde-Ingenieur 52 (1998), p. 43-64
ISSN: 0015-010X
BibTeX: Download
, , , , :
Mobile Multimediakommunikation über DECT, ISDN und LAN
In: Der Fernmelde-Ingenieur 52 (1998), p. 65-74
ISSN: 0015-010X
BibTeX: Download
, , , :
Reduction of Ringing Noise in Transform Image Coding Using a Simple Adaptive Filter
In: Electronics Letters 34 (1998), p. 2110-2112
ISSN: 0013-5194
BibTeX: Download
:
Objektbasierte Codierung von bewegungskompensierten Prädiktionsfehlerbildern in MPEG-4
2. ITG-Fachtagung Codierung für Quelle, Kanal und Übertragung, ITG-Fachbericht 146 (Aachen, 3. March 1998 - 5. March 1998)
BibTeX: Download
, :
Complexity and PSNR-Comparison of Several Fast Motion Estimation Algorithms for MPEG-4
Applications of Digital Image Processing XXI, SPIE (San Diego, 21. July 1998 - 24. July 1998)
BibTeX: Download
, , , , , , , :
MPEG-4 for Broadband Communications
Multimedia Systems and Applications, SPIE (Boston, Mass., 2. November 1998 - 4. November 1998)
BibTeX: Download
, , , , , :
1997
Mobile Multimediakommunikation über DECT, ISDN und LAN
Multimedia: Anwendungen, Technologie, Systeme, ITG-Fachbericht 144 (Dortmund, 29. September 1997 - 1. October 1997)
BibTeX: Download
, , , :
Adaptive constrained least squares restoration for removal of blocking artifacts in low bit rate video coding
IEEE International Conference on Acoustics, Speech, and Signal Processing (Munich, 21. April 1997 - 24. April 1997)
BibTeX: Download
:
Adaptive Low-Pass Extrapolation for Object-Based Texture Coding of Moving Video
Visual Communications and Image Processing, SPIE (San José, 12. February 1997 - 14. February 1997)
BibTeX: Download
:
On the Performance of the Shape Adaptive DCT in Object-Based Coding of Motion Compensated Difference Images
Picture Coding Symposium, ITG-Fachbericht 143 (Berlin, 10. September 1997 - 12. September 1997)
BibTeX: Download
, :
Reduction of Block Artifacts by Selective Removal and Reconstruction of the Block Borders
Picture Coding Symposium, ITG-Fachbericht 143 (Berlin, 10. September 1997 - 12. September 1997)
BibTeX: Download
, , :
1996
Improving the Image Quality of Blockbased Video Coders by Exploiting Interblock Redundancy
First International Workshop on Wireless Image/Video Communications, Loughborough (, 4. September 1996 - 5. September 2010)
BibTeX: Download
, , :
Quality Improvement of Low Data-rate Compressed Video Signals by Pre- and Postprocessing
Digital Compression Technologies and Systems for Video Communications, Berlin (, 7. October 1996 - 9. October 1996)
BibTeX: Download
, , :
1994
Efficient Prediction of Uncovered Background in Interframe Coding Using Spatial Extrapolation
IEEE International Conference on Acoustics, Speech, and Signal Processing, Adelainde (, 19. April 1994 - 22. April 1994)
BibTeX: Download
, :
Uncovered Background Prediction in an Object-Oriented Coding Environment
International Workshop on Coding Techniques for Very Low Bit-rate Video, Colchester (, 7. April 1994 - 8. April 1994)
BibTeX: Download
, :
1993
Region-Based Image Coding Using Functional Approximation
Picture Coding Symposium, Lausanne (, 17. March 1993 - 19. March 1993)
BibTeX: Download
, :
1991
DSP-Based Compression of Four-Color Printed Images
International Conference on DSP Applications and Technology, Berlin (, 28. October 1991 - 31. October 1991)
BibTeX: Download
, :
Variable Blocksize Transform Coding of Four-Color Printed Images
Visual Communication and Image Processing, Boston, Mass., SPIE (, 11. November 1991 - 13. November 1991)
BibTeX: Download
, :